Maximizing Efficiency with Semantic Segmentation Labeling Tools
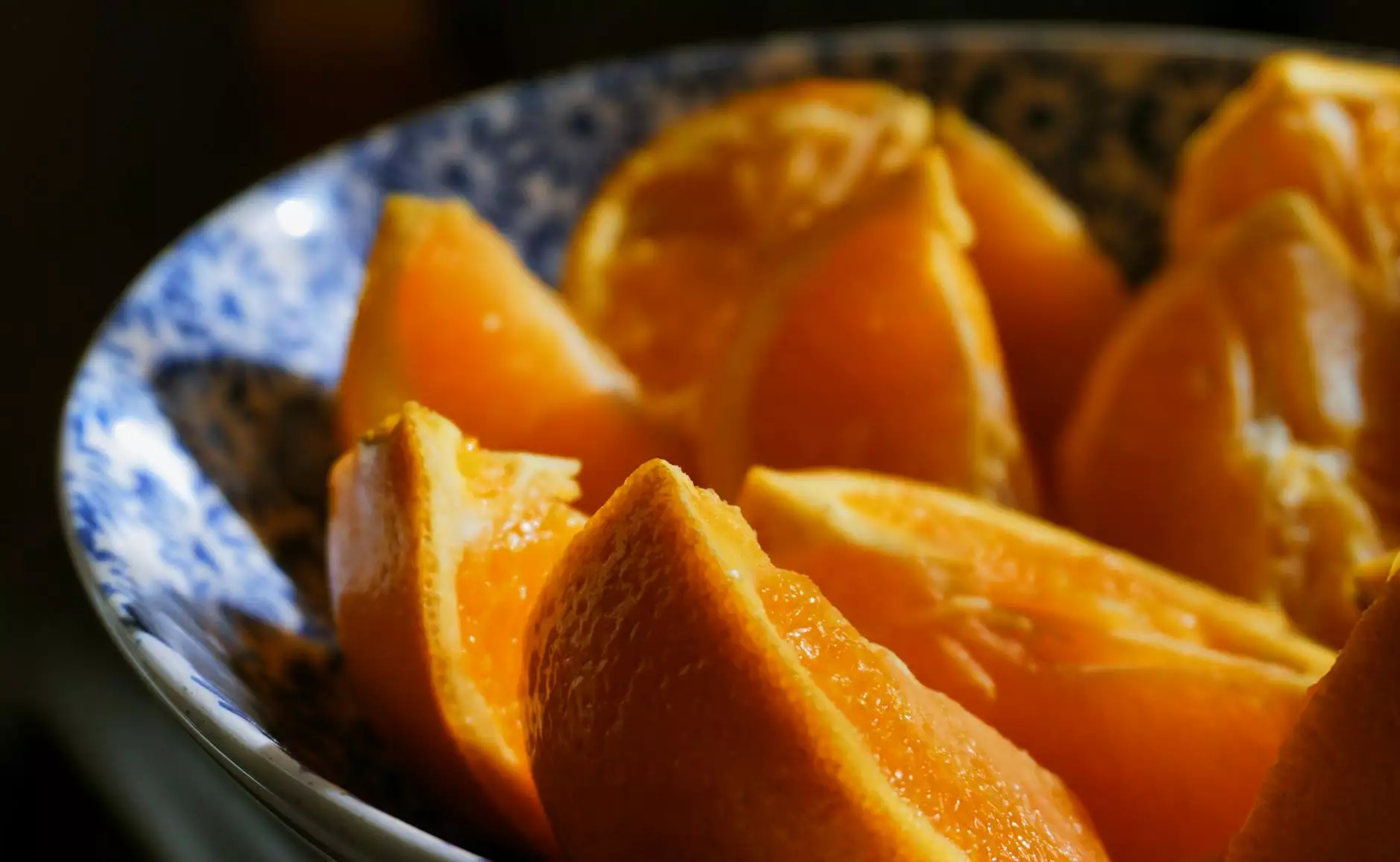
Understanding Semantic Segmentation
Semantic segmentation is a crucial technique in the field of computer vision that classifies each pixel in an image into predefined categories. This process allows machines to understand an image in a highly granular way, which is essential for tasks such as image recognition, autonomous driving, medical imaging, and robotics. By assigning labels to each pixel, semantic segmentation provides a more detailed understanding of an image compared to traditional classification methods.
Importance of Data Annotation in Semantic Segmentation
In the realm of artificial intelligence, particularly in deep learning models, the quality of the data used to train these models significantly impacts their performance. Data annotation refers to the process of labeling raw data, which is vital for teaching machine learning algorithms how to interpret and classify new data accurately. When it comes to semantic segmentation, the need for accurate and detailed annotations becomes even more pronounced. Here are some key points regarding the importance of data annotation:
- Improved Accuracy: High-quality labeled data leads to better-performing models.
- Reduced Bias: Comprehensive annotation practices help mitigate bias in AI algorithms.
- Increased Efficiency: Effective annotation tools streamline the data labeling process, saving time and resources.
What is a Semantic Segmentation Labeling Tool?
A semantic segmentation labeling tool is designed specifically to assist data scientists and machine learning practitioners in annotating their datasets with pixel-wise accuracy. Unlike traditional labeling tools, which may only allow for bounding boxes or regions of interest, semantic segmentation tools provide the functionality to label each individual pixel in an image. This enhances the model’s ability to learn complex patterns and directions within images.
Key Features of a Semantic Segmentation Labeling Tool
When selecting a semantic segmentation labeling tool, it’s essential to consider various features that can enhance the labeling process. Here are some of the critical features to look for:
- Intuitive User Interface: A user-friendly interface allows annotators to label images quickly and accurately.
- Advanced Annotation Capabilities: Support for polygonal or brush tools enables more detailed annotations.
- Integration with Machine Learning Pipelines: The ability to seamlessly integrate with existing workflows enhances productivity.
- Collaboration Features: Tools that allow teams to collaborate in real-time can improve workflow efficiency.
- Quality Assurance Mechanisms: Built-in quality checks and review processes can ensure the integrity of the labeled data.
Benefits of Using Semantic Segmentation Labeling Tools at Keylabs.ai
At keylabs.ai, we offer a cutting-edge semantic segmentation labeling tool designed to optimize data annotation processes for various industries. Here’s why you should consider our platform:
- High Precision: Our tool ensures consistent and precise labeling, which is critical for training AI models.
- Time-Saving Features: Automated suggestions and shortcut keys can significantly reduce the time taken for data preparation.
- Flexible Pricing Models: We cater to both startups and large enterprises with scalable solutions.
- Comprehensive Support: Our dedicated support team is available to assist you throughout your data annotation journey.
Applications of Semantic Segmentation
Semantic segmentation has a broad range of applications across different industries. Here are some notable examples:
1. Autonomous Vehicles
In the automotive industry, semantic segmentation plays a pivotal role in helping self-driving cars to identify pedestrians, road signs, lane boundaries, and other vehicles, thereby enhancing safety and navigation capabilities.
2. Medical Imaging
In healthcare, semantic segmentation is utilized to accurately delineate structures within medical images such as MRI scans and CT scans, facilitating better diagnosis and treatment planning.
3. Agriculture
Agricultural technology utilizes semantic segmentation to analyze crops from aerial images, helping farmers in monitoring plant health, optimizing yield, and making data-driven decisions.
4. Video Surveillance
In security systems, semantic segmentation is used to enhance the capabilities of surveillance cameras to identify unusual behaviors or detect intruders by analyzing scene context.
Challenges in Semantic Segmentation and Overcoming Them with Keylabs.ai
While semantic segmentation offers numerous benefits, it also poses several challenges that organizations face:
- Data Imbalance: Some categories may have significantly fewer examples, leading to biased models.
- Labeling Complexity: Accurately labeling images can be time-consuming and requires skilled annotators.
- Quality Control: Maintaining high-quality annotations across large datasets can be difficult.
At keylabs.ai, we tackle these challenges head-on by:
- Implementing Advanced Algorithms: Our tool uses AI-driven methods to suggest labels and streamline the annotation process.
- Providing Training and Support: We offer training for your team to enhance their skills in data annotation.
- Establishing Review Workflows: Our platform includes quality control measures to ensure the accuracy of annotations.
The Future of Semantic Segmentation
The field of semantic segmentation is continually evolving, and its potential applications are expanding rapidly. With advancements in deep learning and computer vision technologies, we can expect:
- Improved Algorithms: Ongoing research will lead to more sophisticated models that require less annotated data.
- Greater Accessibility: Tools and datasets will become more accessible to a broader audience, democratizing AI development.
- Integration with Augmented Reality (AR): Semantic segmentation is expected to enhance AR experiences by improving object recognition in real-time environments.
Conclusion
In conclusion, the use of a semantic segmentation labeling tool is essential for businesses looking to leverage the power of AI and machine learning. At keylabs.ai, we are committed to providing robust solutions that enhance the data annotation process, driving efficiency and accuracy in machine learning projects. Embrace the future of data annotation and optimize your workflows today!