The Significance of Labeled Image Datasets in Modern Business
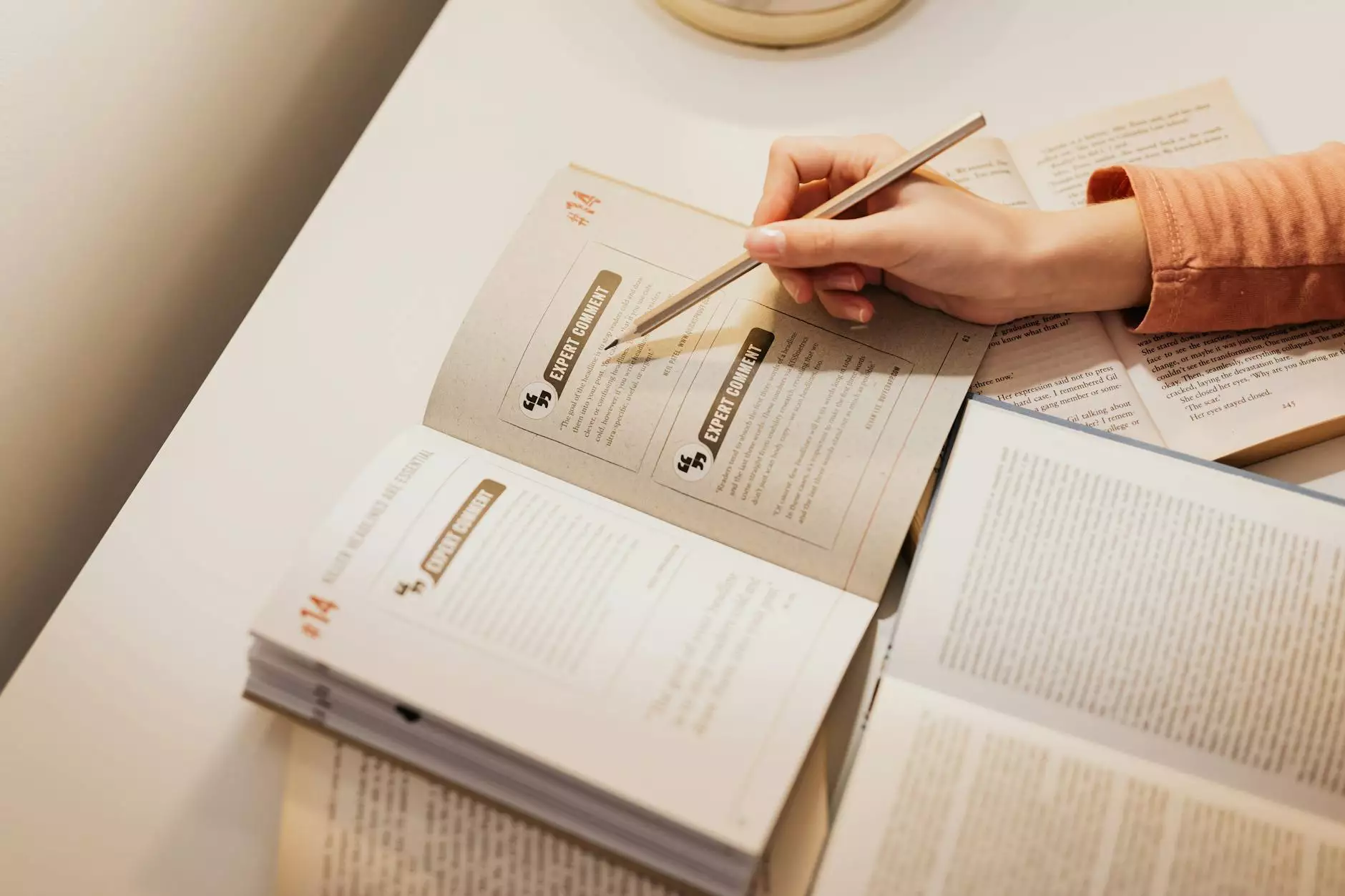
In today's rapidly advancing technological landscape, labeled image datasets have emerged as a critical asset for businesses looking to leverage artificial intelligence (AI) and machine learning (ML). These datasets serve as the backbone for training algorithms, enabling machines to interpret and recognize visual data. As we delve into the world of data annotation tools and platforms, we'll uncover how these resources are transforming industries and improving operational efficiency.
Understanding Labeled Image Datasets
Labeled image datasets consist of images that are tagged or annotated with relevant information, allowing machines to understand the content within those images. This might include identifying objects, contexts, or even sentiments expressed within an image. For instance, a dataset of traffic signs would have images of various signs along with their corresponding labels, such as "Stop Sign," "Yield Sign," or "Speed Limit." The comprehensive nature of these datasets enables AI systems to learn and improve exponentially over time.
The Role of Data Annotation Tools
Data annotation tools are essential in the creation of labeled image datasets. They facilitate the process of tagging and organizing data so that it can be effectively utilized in AI and ML models. Here's a closer look at the key features of data annotation tools:
- User-Friendly Interface: Modern annotation tools offer intuitive interfaces that allow users to annotate images with ease, minimizing the time spent on training annotators.
- Variety of Annotation Types: These tools support multiple annotation types, such as bounding boxes, polygons, and semantic segmentation, ensuring comprehensive data labeling.
- Collaboration Features: Many data annotation platforms enable teams to collaborate seamlessly, allowing for real-time updates and feedback during the annotation process.
- Quality Assurance: Advanced tools incorporate quality assurance mechanisms to ensure the accuracy and consistency of annotations, which is pivotal for maintaining dataset integrity.
Benefits of Using Labeled Image Datasets
Utilizing labeled image datasets brings a multitude of benefits to businesses across various sectors. Here are some critical advantages:
1. Enhanced Machine Learning Models
The accuracy of machine learning models heavily depends on the quality of the training data. By using labeled image datasets, businesses can significantly enhance their model's performance, leading to better predictions and outcomes. For example, in the healthcare sector, labeled datasets of medical images allow AI to assist in diagnosing ailments with remarkable precision.
2. Cost-Effective Solutions
Data annotation can be a labor-intensive process, but leveraging automated tools can drastically reduce costs. By investing in a reliable data annotation platform, businesses can speed up the process of creating labeled datasets, thus saving both time and financial resources.
3. Scalability
As businesses grow, so do their data needs. Labeled image datasets facilitate scalability, allowing organizations to expand their data collections and utilize them efficiently in various projects without significant hurdles. This adaptability is crucial for tech companies and startups aiming to remain competitive in a fast-paced environment.
Data Annotation Platforms: Key Players in the Industry
Several prominent data annotation platforms have risen in popularity, offering sophisticated solutions to businesses. Here are a few notable providers:
- Keylabs.ai: Specializing in high-quality data annotation, Keylabs.ai provides a range of services that ensure precise and reliable labeled datasets. Their platform supports various annotation techniques, and they employ a team of experienced annotators for optimal results.
- Scale AI: Known for its flexibility and robustness, Scale AI provides top-tier data annotation services for various applications, including autonomous vehicles and computer vision tasks.
- Labelbox: A user-friendly platform designed for fast and efficient data labeling, Labelbox integrates collaboration tools to enhance team productivity during the data annotation process.
Real-World Applications of Labeled Image Datasets
Labeled image datasets are not just theoretical; they are utilized across various sectors. Let's explore some key applications:
1. Autonomous Vehicles
In the automotive industry, labeled image datasets play a pivotal role in developing autonomous driving technologies. By leveraging these datasets, AI systems learn to recognize road signs, pedestrians, and obstacles, which is essential for safe navigation.
2. Retail and E-Commerce
Online retailers use labeled image datasets to enhance product recognition systems. By tagging images with product details, businesses can improve search functionalities and personalized recommendations for users, leading to higher conversion rates.
3. Medical Imaging
The healthcare sector benefits immensely from labeled datasets in diagnostics. AI algorithms trained on annotated medical imaging data can identify conditions such as tumors or fractures, assisting doctors in making more informed decisions.
4. Agriculture
Farmers and agribusinesses utilize labeled image datasets for crop monitoring and pest detection. With accurate data annotation, AI systems can analyze images to identify plant health, assess soil quality, and predict yields, leading to smarter farming practices.
Challenges in Data Annotation
Despite the numerous benefits, data annotation is not without its challenges. Some of the primary hurdles include:
- Quality Control: Ensuring the accuracy and consistency of annotations can be difficult, especially with large datasets.
- Time Consumption: Creating labeled datasets can be a time-consuming process, particularly for complex tasks that require human insight.
- Cost Implications: While automated tools help reduce costs, high-quality annotations often require significant financial investment, particularly in specialized domains.
The Future of Labeled Image Datasets
The landscape of labeled image datasets is continuously evolving, and several trends are shaping their future:
1. Increased Automation
As AI technology advances, automation in data annotation will become more prevalent. Automated annotation tools are anticipated to evolve, making the process faster and more efficient. This will likely reduce dependency on human annotators, although human oversight will remain essential for maintaining quality.
2. Enhanced Collaborative Platforms
The demand for collaboration among teams is growing, pushing data annotation platforms to innovate. Enhanced features that allow for real-time collaboration and feedback will become standard, promoting efficiency and quality in annotations.
3. Integration with AI Workflows
Many businesses will seek to integrate data annotation tools into their AI development workflows seamlessly. This will allow for a more streamlined approach to data processing, ensuring that labeled datasets can be rapidly iterated and improved upon.
Conclusion
In summary, labeled image datasets are essential for businesses looking to thrive in the era of AI and machine learning. By investing in robust data annotation tools and platforms, organizations can create high-quality datasets that improve their models' performance and accelerate innovation. As the industry evolves, staying ahead of these trends will be critical for businesses aiming to leverage the full potential of AI.
With platforms like Keylabs.ai leading the way, companies can ensure they have access to the best labeled image datasets available, empowering them to achieve remarkable results in their respective fields.